Milestones in Facial Recognition Technology
Facial recognition technology (FRT) has rapidly transformed from a speculative idea into a ubiquitous feature of modern life, playing a significant role in security, commerce, and personal devices.
From its early theoretical foundations to the sophisticated AI-driven systems we see today, the history of facial recognition is a fascinating journey through technological and scientific advancements.
This article provides an overview of key milestones that have shaped the development of FRT.
Do you need help redacting personal data from your videos and staying compliant?
Submit your email to get in touch with our team about video redaction by heads, bodies, license plates, and using customisable shapes.
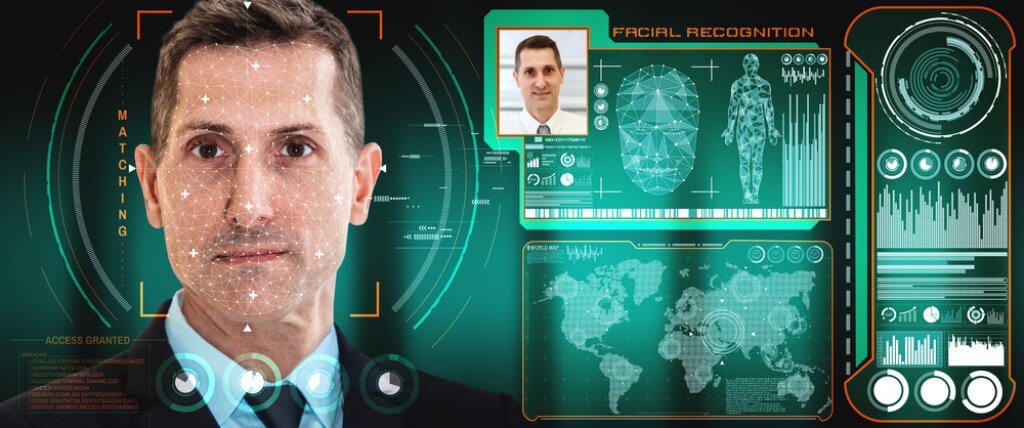
1. Early Concepts and Foundations (1960s–1970s)
The concept of automating facial recognition was explored as early as the 1960s. During this time, scientists and researchers began investigating how to create algorithms that could identify human faces from images.
One of the early pioneers, Woody Bledsoe, is widely regarded as a founder of this technology. He, along with Helen Chan Wolf and Charles Bisson, developed semi-automated systems that required humans to manually locate facial features like the eyes, ears and nose. These coordinates were then fed into a computer that would attempt to match them to stored images.
This initial stage laid the groundwork for future developments by demonstrating that faces could be numerically represented, although the technology still relied heavily on human input.
2. Eigenfaces and the Rise of Algorithms (1980s–1990s)
In the 1980s, facial recognition technology made a significant leap with the introduction of mathematical approaches to pattern recognition. One of the most influential breakthroughs came in 1988 when Sirovich and Kirby developed a method that could efficiently represent faces using principal component analysis (PCA). This method, known as Eigenfaces, was revolutionary for its ability to reduce the complexity of facial images and identify key features that distinguished one face from another.
In 1991, Matthew Turk and Alex Pentland at MIT expanded on this work by demonstrating how Eigenfaces could be used for real-time facial recognition.
This represented a major step forward, as the algorithm could now work without manual intervention and was capable of recognising faces in a more automated manner.
3. Facial Recognition Enters the Mainstream (2000s)
By the early 2000s, facial recognition technology began to see practical applications, particularly in law enforcement and security.
The U.S. Department of Defense’s Face Recognition Technology (FERET) Program, initiated in 1993, aimed to develop a standardised method for evaluating facial recognition algorithms. This program significantly advanced the field by creating a large, publicly available dataset for training and testing algorithms, which helped to drive innovation.
In 2001, facial recognition technology gained international attention when it was used during the Super Bowl to scan the faces of attendees for potential criminals. Although this deployment generated controversy over privacy concerns, it highlighted the growing potential for FRT in large-scale security applications.
Around this time, companies developed commercial facial recognition systems for government agencies, airports and casinos.
These systems continued to improve in accuracy, but still struggled with challenges like lighting variations, occlusion and aging.
4. The AI Revolution and Deep Learning (2010s)
The 2010s marked the beginning of a new era for facial recognition technology, thanks to advances in machine learning and artificial intelligence (AI). Specifically, the development of convolutional neural networks (CNNs) transformed the field by enabling computers to learn how to identify faces in a more flexible and robust way. These networks, capable of processing large amounts of visual data, made facial recognition far more accurate and adaptable.
In 2014, DeepFace, an AI system developed by Facebook, set a new benchmark by achieving a 97% accuracy rate in facial recognition tasks - nearly on par with human performance. Around the same time, Google introduced its own system, FaceNet, which further improved accuracy and efficiency. These systems could now reliably identify faces in a variety of conditions, including low lighting and different angles, which represented a significant improvement over earlier methods.
Moreover, the availability of large datasets like Labelled Faces in the Wild (LFW) and advances in computational power enabled researchers to train deep learning models more effectively, which led to widespread adoption in commercial applications.
5. Widespread Adoption and Ethical Concerns (Late 2010s–2020s)
As facial recognition technology became more accurate and accessible, its use expanded across numerous sectors.
Smartphones, with Apple's introduction of Face ID in 2017, brought FRT to millions of users, making face unlocking a popular feature. Similarly, facial recognition became a key tool in public security, especially in large urban centres and airports.
However, the rapid spread of FRT has also sparked ethical concerns, particularly around privacy, bias and surveillance.
Studies have shown that early facial recognition systems often exhibited racial and gender bias, which raises questions about fairness and the potential for misuse. For instance, a 2018 MIT study found that commercial facial recognition systems had much higher error rates when identifying darker-skinned individuals and women.
In response, governments and regulatory bodies began exploring policies to control the use of FRT. Some cities, like San Francisco and Boston, have banned the use of facial recognition by law enforcement, while others have implemented stricter guidelines to ensure its ethical use.
6. Current Trends and the Future of Facial Recognition (2020s and Beyond)
In the 2020s, facial recognition technology continues to evolve, driven by advancements in AI, increased computational power and a growing body of research on reducing bias and improving accuracy.
New applications are emerging, from personalised marketing in retail to enhanced security in financial services.
At the same time, the future of facial recognition will depend heavily on navigating ethical challenges. Issues like mass surveillance, consent and data protection remain at the forefront of the conversation. Researchers and policymakers are working together to develop more transparent, fair and privacy-respecting solutions.
Despite privacy and fairness concerns, facial recognition technology is poised to remain a key component of the digital landscape, shaping everything from security protocols to personal device interactions.
With ongoing developments in AI and machine learning, the accuracy and utility of facial recognition will continue to grow, which will potentially unlock new possibilities that have yet to be imagined.
Facial Recognition Technology Privacy Concerns
Facial recognition technology, while useful for security and identification, raises significant ethical concerns around privacy and data protection. Central to these concerns is the potential for misuse of sensitive biometric data, such as the unauthorised storage or sharing of facial data by corporations or governments. Facial recognition often requires extensive image processing and can inadvertently capture and analyse individuals without their consent, which infringes on their right to privacy.
Furthermore, data protection regulations, such as GDPR, mandate strict protocols for handling personal data, emphasising transparency, consent and the right to be forgotten. However, facial recognition often operates in public spaces, which complicates consent acquisition and makes it challenging to enforce user control over their data. Additionally, biased algorithms can lead to unfair treatment, especially for minority groups, underscoring the need for transparency and accountability in algorithm design and deployment.
Balancing the benefits of facial recognition with ethical data protection practices is essential to safeguard individual rights.
Conclusion
The evolution of facial recognition technology reflects the broader trends in computer science and artificial intelligence.
From early manual systems in the 1960s to today's AI-driven algorithms, each milestone has brought the technology closer to its full potential.
While the future of FRT holds immense promise, it also requires thoughtful consideration of its ethical implications to ensure that its benefits are realised in a way that respects individual rights and societal norms.
Facit Redaction Solutions for Ethical Video Processing
Advances in deep learning have significantly enhanced the capabilities of facial recognition technology, and Facit stands at the forefront of these innovations.
Leveraging improved algorithmic efficiency, Facit delivers highly accurate, real-time facial recognition solutions that prioritise privacy and data security.
Facit’s models are trained on diverse datasets, which reduces bias and improves accuracy across various demographics.
Facit's cutting-edge approach also emphasises ethical AI by incorporating transparency and fairness into their design.
Managing FRT and data privacy ethically and effectively enables organisations to implement CCTV security measures and maintain the trust of the public.
Facit’s Identity Cloak eliminates FRT privacy concerns by masking the identities of all but the subject(s) of interest before video footage is shared with third parties.
FAQs: Facial Recognition Technology
1. What is facial recognition technology?
Facial recognition uses algorithms to analyse and identify human faces in images or videos, matching them against stored data for verification or identification.
2. How does FRT work?
The technology maps facial features, creating a "faceprint" that can be compared with databases to identify individuals.
3. Is facial recognition safe?
While useful, FRT raises privacy and security concerns, as unauthorised data storage or use can violate personal privacy rights.
4. Where is FRT used?
Applications include security, law enforcement, unlocking devices and personalized marketing.
5. Are there ethical concerns?
Yes, including data privacy, consent, bias and potential misuse of personal information.
Related Articles
Get in touch
Fill in the form below so we can understand your Video Redaction or Analytics requirements.